Recruitments little secret, only handful of resumes are read - is current technology to simple?
Most of us from the recruitment industry, would agree that ímostí resumes are not read.
Why is this the case? Instead of trying to answer question - (there are many write-ups on that topics on WEB) main industry concern is probably: How do we know that we are reading right resume at the right time?
By reading discussions on the WEB seems to be that we all assumed ístatus quoí - neither technology nor recruitment process will evolve. We will be stuck forever with íkeywordsí (simple technology thrown to solve complex problem of resume and job matching) and ívolumesí whereby Job Boards are pure classified business with íemail forwarding functioní.
In a longer text below (apologies), I am trying to place some light on technology evolution aspect, leaving ívolumeí problem for some other discussion....
There are many day-to-day questions of interest in Recruitment:
What is the supply (or demand) for a given position or candidate?
What is the natural career path for a given individual?
What skills would most improve an individualís market value?
How compatible is a given candidate with a given position?
Which of my employees is most likely to leave for another position?
The list goes on. All too often, these questions are not asked until it is too late, and even when they are asked, the answers are frequently based on an ad-hoc analysis of relevant data (e.g., job-postings, resumes, employment-market survey reports, etc.), occasionally with the help of relatively simple tools (i.e keywords).
However, the data to address these specific questions, at the individual level, exists. What has not been available, until now, is the sophisticated technology necessary to extract knowledge from this data, and to present results in a usable way. Effective application of technology can greatly improve the efficiencies in the employment markets.
The technology best suited for these applications is in some ways similar to that used in other industries (credit risks, fraud risks etc) but in other ways unique (more complex) for Recruitment.
Few AI vendors have pioneered the use of Predictive Matching to create an infrastructure for understanding the employment markets and the entities within it, and addressing specific questions of interest, such as finding the best probable matches between candidates and positions.
Predictive Matching technology brings together two areas of AI that have not been combined in the past:
Predictive Modeling/Matching.
Predictive modeling provides a powerful and general framework to make specific forecasts or predictions at the individual level, based on available data about the individuals or entities of interest (like resumes and job description).
In the employment markets, one may wish to build a predictive model that can assess the compatibility of candidate/position pairs.
Statistical Natural Language Processing (SNLP)
Predictive modeling has traditionally been applied in settings where the underlying data is numerical. In the context of Recruitment, much of the relevant data to be understood exists in the form of free, or at best semi-structured, text. For example, in trying to match candidates with positions, candidates may be represented by resumes, and positions by job-postings. With natural language processing techniques, we can extract relevant information from text documents for use in predictive models.
An important potential misconception should be addressed before proceeding further discussion of Predictive Matching technology applied on Recruitment: technology by itself does not make a solution.
Indeed, simply throwing technology at a problem is a recipe for failure. I believe that there are three critical components to developing solutions that effectively address problems in Recruitment: advanced technology; solid domain understanding; and knowledge of operational settings (process).
Technology is an enabler, but it must be married with an understanding of the target domain in order to create a solution focused on the specific problems at hand. An all-terrain Range Rover will do poorly in a Formula 1 car race; similarly, a general web-search tool will not optimally retrieve candidates for a given job position.
This is why horizontal technologies (like keywords) are not in and of themselves a panacea, particularly in a setting where one is attempting to address a specific problem. But domain understanding combined with overly simple technology is just as sub-optimal. The combination of advanced technology (AI) with domain focus is necessary. By no means I am advocating here that AI is ífinal answerí, rather I am trying to make point that AI seems to be next step in a major technological improvement within Recruitment domain.
The third critical element, while neither as sexy as technology nor as obvious as domain understanding, is the ability to design a solution that works in an operational setting.
So, while our focus is on Predictive Matching technology for Recruitment, the importance of domain and application specific knowledge, and overall system design, cannot be overlooked. To succeed, one need all of technology, domain focus, and engineering it can get.
Davor Miskulin
Director Resumo Pty Ltd
Australia
dmiskulin@resumo.com.au
Recruitments little secret, only a handful of resumes are read
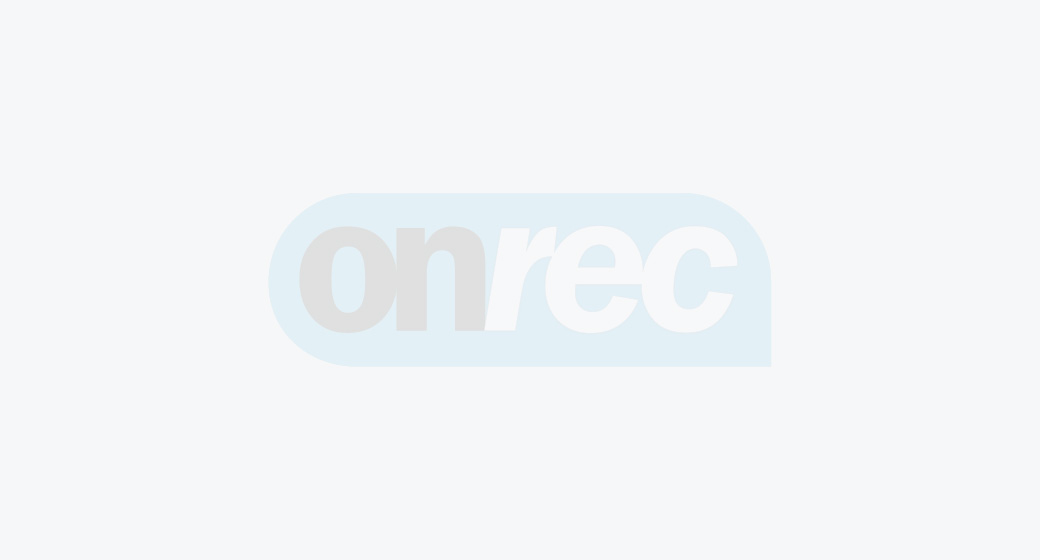
Davor Miskulin Director Resumo Pty Ltd